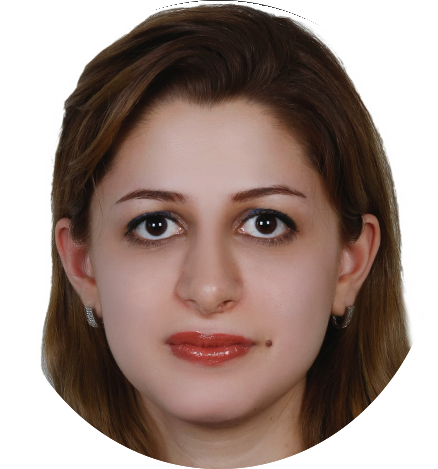
I have been working on analyzing RNA-seq data of soybean developing embryos for the past 3 years. I have developed a computational pipeline for analysis of this RNA-seq data that has a potential to be applied to any species with known reference genome. I have developed and used several algorithms and tools for mining of this time-course RNA-seq data. In addition to finding differentially expressed genes, I have analyzed differentially expressed splice variants of known genes leading to investigation of alternative splicing events such as exon skipping, intron retention, and antisense transcripts. We have further analyzed these splice variants regarding to their domain composition, coding potential, signaling and metabolic pathways, and co-expression network.
Particularly, I am interested in long noncoding RNAs (lncRNAs) and long intergenic RNAs (lincRNAs). lncRNAs and lincRNAs are regulatory RNAs that their functions have been implicated in many diseases such as cancer. For this purpose, I have developed an SVM classifier with over 98% accuracy that can classify transcripts into coding and noncoding classes with integration of several features related to sequence and structure of a transcript. This SVM classifier is currently trained, tested, and applied on Arabidopsis and soybean transcripts. I am going to extend this classifier to human, mouse, zebrafish, and Drosophila.
We have detected all transcripts that were expressed during soybean embryo development. These transcripts include known transcripts, intergenic transcripts, and novel splice variants of known genes. We have investigated all these transcripts for their coding potential, and their domains.
We have detected 10,798 genes that changed significantly at least in one of the time-points. We have studied these genes using gene ontology enrichment analysis and MapMan metabolic pathway analysis.
Developing soybean seeds accumulate oils, proteins, and carbohydrates that are used as oxidizable substrates providing metabolic precursors and energy during seed germination. The accumulation of these storage compounds in developing seeds is highly regulated at multiple levels, including at transcriptional and post-transcriptional regulation. RNA sequencing was used to provide comprehensive information about transcriptional and post-transcriptional events that take place in developing soybean embryos. Bioinformatics analyses lead to the identification of different classes of alternatively spliced isoforms and corresponding changes in their levels on a global scale during soybean embryo development. Alternative splicing was associated with transcripts involved in various metabolic and developmental processes, including central carbon and nitrogen metabolism, induction of maturation and dormancy, and splicing itself. Detailed examination of selected RNA isoforms revealed alterations in individual domains that could result in changes in subcellular localization of the resulting proteins, protein-protein and enzyme-substrate interactions, and regulation of protein activities. Different isoforms may play an important role in regulating developmental and metabolic processes occurring at different stages in developing oilseed embryos.
Emergence of next generation sequencing has revealed significant levels of transcriptional activity within both unannotated and annotated regions of the genome, leading to construction of novel tramscripts. These novel transcripts may be located in the genic regions such as antisense, overlapped intronic, and overlapped exonic or may be located in the intergenic regions. However, they can be coding or noncoding in the broader aspect. Hence, one of the main tasks is to functionally characterize these novel transcripts and to determine if they are coding or noncoding (ncRNA). Although the functions of coding genes have been studied for many years, trends to characterize the function of noncoding transcripts have been started recently. Several evidences for implication of ncRNAs in control of development, growth, and disease have been reported so far. ncRNAs can perform their functions through different mechanisms such as chromatin modifications (epigenetic control of gene expression), RNA-protein interactions, and transcriptional inference.
We propose a support vector machine classifier, which can classify novel transcripts into coding or noncoding with over 96% accuracy. Several sequential and structural features have been compiled for training the classifier. The classifier has been used to classify the novel assembled transcripts from RNA-sequencing pipelines for Soybean and Arabidopsis organisms. However, it can be adapted to any species.
The recent ample availability of Chromatin Immunoprecipitation Sequencing (ChIP-Seq) data presents a distinct opportunity to study the action of transcription factors, particularly in plant science. Even though in vivo transcriptional regulation often involves the concerted action of more than one transcription factor, the format of each individual ChIP-Seq dataset usually represents the action of only one transcription factor. Therefore, a relational database in which all available ChIP-Seq datasets are curated is essential and necessary.
We present Expresso (database and webserver) as a tool for collection and integration of all available Arabidopsis ChIP-Seq data, which in turn can be linked to a user’s gene expression data. Known target genes of transcription factors were identified by motif analysis on publicly available ChIP-Seq data in GEO DataSets. Expresso currently provides three services for identifying: 1) the target genes of a given transcription factor, 2) the transcription factors that regulate a gene of interest, 3) the correlation of gene expression between transcription factors and their target genes.
Expresso is currently under development.
Check out Expresso here!Much of the cellular activity is organized as a network of interacting modules, which are the set of genes interacting together in different conditions to make the cell responsive to different stresses and conditions in the environment as well as inside the cell. Recently, there has been much interest in reverse engineering these networks using gene expression data. One of the popular methods for reverse engineering of these regulatory networks using gene expression data is using Bayesian networks.
Gene expression data by itself suffers from high noise and lack of enough power for inferring causal relationship between genes. Incorporating prior biological knowledge can improve performance and can help to produce more biologically meaningful network. Current project: The goal is to learn a Bayesian network for selected soybean genes from time series gene expression data of soybean developing embryos produced by high throughput sequencing technique (RNA-seq). We take advantage of multiple data types, including co-expression and co-localization for interaction between Arabidopsis homologs of soybean genes as prior knowledge by incorporating these data into gene expression data for learning structure of Bayesian network using Markov Chain Monte Carlo (MCMC) structure learning algorithm.